Quote
: |
刘伟,邹伟红,卢彦杰,胡为,刘塔斯.基于迁移学习和模型融合的中药材图像识别研究[J].湖南中医药大学学报英文版,2022,42(5):809-814.[Click to copy
] |
|
|
|
This paper
:Browser 2142times Download 579times |
基于迁移学习和模型融合的中药材图像识别研究 |
刘伟,邹伟红,卢彦杰,胡为,刘塔斯 |
(湖南中医药大学信息科学与工程学院, 湖南 长沙 410208;湖南中医药大学药学院, 湖南 长沙 410208) |
摘要: |
目的 为了提高中药材图像识别的准确率,针对中药材形状不规则、纹理特征细微、种类繁多等特点,结合深度学习方法开展中药材图像识别研究。方法 通过爬虫获取中药材图像并进行数据预处理,构建中药材图像数据集,使用Xception、DenseNet作为主干网络提取中药材特征,通过迁移学习、数据增强、模型融合等方法优化网络结构,并提出一种基于Xception和DenseNet融合的中药材图像识别模型DxFusion。结果 通过迁移学习、数据增强和模型融合,DxFusion在60种中药材测试集上分类精度可达99.65%,效果优于已有分类模型。结论 基于迁移学习和多模型融合的深度卷积神经网络可以提取图像中不规则的中药材特征,有效提升中药材图像识别的准确率。 |
关键词: 深度学习 中药材识别 卷积神经网络 迁移学习 数据增强 模型融合 |
DOI:10.3969/j.issn.1674-070X.2022.05.019 |
Received:October 26, 2021 |
基金项目:湖南省重点研发计划项目(2018SK2112);湖南省教育厅科学研究项目(20C1435)。 |
|
Research on image recognition of Chinese medicinal materials based on transfer learning and model fusion |
LIU Wei,ZOU Weihong,LU Yanjie,HU Wei,LIU Tasi |
(School of Information Science and Engineering, Hunan University of Chinese Medicine, Changsha, Hunan 410208, China;School of Pharmacy, Hunan University of Chinese Medicine, Changsha, Hunan 410208, China) |
Abstract: |
Objective In order to improve the accuracy of Chinese medicinal materials image recognition, the research of Chinese medicinal materials image recognition is carried out based on deep learning according to the characteristics of irregular shape, subtle texture characteristics, and various types. Methods Images of Chinese medicinal materials were obtained through crawlers and data preprocessing was performed, image data sets of Chinese medicinal materials were established, Xception and DenseNet was used as the backbone network to extract the characteristics of Chinese medicinal materials, and the network structure was optimized by transfer learning, data augmentation and model fusion. A method called DxFusion for image recognition of Chinese medicinal materials based on the fusion of Xception and DenseNet was proposed. Results Through transfer learning, data augmentation and model fusion, the classification accuracy of DxFusion was reached 99.65% on 60 kinds of Chinese medicinal materials test sets, which was better than the existing classification models. Conclusion The deep convolutional neural network based on transfer learning and multi-model fusion can extract the irregular characteristics of Chinese medicinal materials in the image, and effectively improve the accuracy for the image recognition of Chinese medicinal materials. |
Key words: deep learning recognition of Chinese medicinal materials convolutional neural network transfer learning data augmentation model fusion |
|
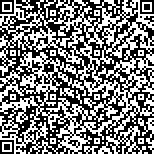 二维码(扫一下试试看!) |
|
|
|
|