引用本文: |
周展,刘彬,郑立瑞,谭建聪,邹北骥,彭清华,肖晓霞.一种面向不平衡数据的心脏病风险预测可解释性框架[J].湖南中医药大学学报,2023,43(6):1078-1085[点击复制] |
|
|
|
本文已被:浏览 1227次 下载 1183次 |
一种面向不平衡数据的心脏病风险预测可解释性框架 |
周展,刘彬,郑立瑞,谭建聪,邹北骥,彭清华,肖晓霞 |
(湖南中医药大学信息科学与工程学院, 湖南 长沙 410208;湖南中医药大学信息科学与工程学院, 湖南 长沙 410208;中南大学计算机学院, 湖南 长沙 410083;湖南中医药大学中医学院, 湖南 长沙 410208) |
摘要: |
目的 研究疾病预测模型存在的类别不平衡性与不可解释性难题。方法 结合极限梯度提升(eXtreme gradient boosting, XGBoost)、混合采样和Shapley加法解释(shapley additive exPlanations, SHAP)分析,提出一种面向不平衡数据的心脏病风险预测可解释性框架ICRPI。结果 该框架下的风险预测模型平衡准确度为0.942 50,AUC为0.986 03,模型可视化分析获得高龄、高体质量指数(body mass index, BMI)值、患有糖尿病等9个心脏病危险因素,并得出高龄的糖尿病患者、高BMI值且诊断为糖尿病或临界糖尿病患者、高BMI值且缺乏体力活动群体为患心脏病高危群体,临界糖尿病人群参与体力活动可降低患心脏病风险。结论 ICRPI框架适用于真实临床不平衡数据分析,且能明确给出致病风险因素及其相关性,可有效提高临床诊断准确率的同时为医生提供致病因素分析,智能辅助医生临床诊疗。 |
关键词: 数据类别不平衡|心脏病风险预测|XGBoost|SHAP|可解释性 |
DOI:10.3969/j.issn.1674-070X.2023.06.019 |
投稿时间:2022-12-03 |
基金项目:科技部十三五重点研发计划项目(2017YFC1703300);科技创新2030“新一代人工智能”重大项目课题(2018AAA0102102);2022年湖南中医药大学研究生创新课题立项基金项目(2022CX123)。 |
|
A framework for predicting heart disease risk factors with interpretability by imbalanced data |
ZHOU Zhan,LIU Bin,ZHENG Lirui,TAN Jiancong,ZOU Beiji,PENG Qinghua,XIAO Xiaoxia |
(School of Informatics, Hunan University of Chinese Medicine, Changsha, Hunan 410208, China;School of Informatics, Hunan University of Chinese Medicine, Changsha, Hunan 410208, China;School of Computer Science and Engineering, Central South University, Changsha, Hunan 410083, China;School of Chinese Medicine, Hunan University of Chinese Medicine, Changsha, Hunan 410208, China) |
Abstract: |
Objective To solve the problems caused by imbalanced data and interpretability of disease prediction models. Methods ICRPI, the framework for predicting heart disease risk factors with interpretability by imbalanced data was proposed by combining eXtreme Gradient Boosting(XGBoost), mixed sampling, and Shapley Additive exPlanations(SHAP). Results The balance accuracy of the risk prediction model within this framework was 0.942 50, and the AUC was 0.986 03. Nine heart disease factors such as older age, high body mass index (BMI) value, and diabetes were obtained by model visualization analysis. The older diabetic patients, the diabetes or borderline diabetes with high BMI value, the patients with high BMI and lacking physical activities are high-risk groups for heart disease; while for the borderline diabetes, physical activity can reduce the risk of heart disease. Conclusion The ICRPI framework can analyze real clinical imbalance data, and can clearly show the pathogenic factors and their correlations. It can effectively improve the accuracy of clinical diagnosis, provide pathogenic factor analysis for doctors, and intelligently assist doctors in clinical practice. |
Key words: imbalanced data|predicting heart disease risk factors|XGBoost|SHAP|interpretability |
|
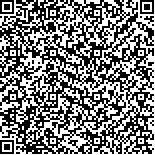 二维码(扫一下试试看!) |
|
|
|
|